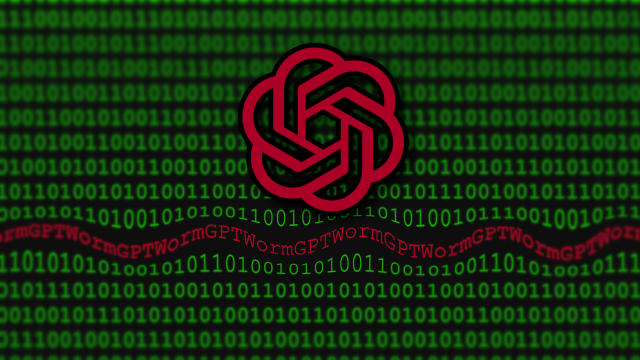
The Risks of Blindly Embracing AI in Enterprises
Artificial Intelligence (AI) has become identified with innovation and progress in the current technological landscape. Many enterprise organizations rush to integrate the technology into their operations simply to help them be categorized among “edge technology adapters.” This enthusiasm usually overlooks the myriad of risks associated with adoption. This article comprehensively looks at these risks while focusing on the importance of a cautious and informed approach to integration.
Privacy Issues
One of the significant concerns with AI-related risks focuses on privacy. To effectively function, AI systems usually handle many data, which is often personal and sensitive. If this data is not collected, stored, and processed with the necessary care, it can lead to severe infringements on privacy. For instance, the use of facial recognition systems for security purposes could inadvertently infringe upon the privacy rights of individuals, hence leading to ethical and legal complications.
Additionally, even from innocuous data, artificial intelligence can infer information of a sensitive nature, which can aggravate concerns over privacy. For instance, social media can be mined by AI algorithms for inferences on personal preferences, health conditions, or even financial status. All that level of insight can lead to undesired profiling and discrimination.
To mitigate these risks, organizations need to take concrete steps to ensure data protection by anonymizing data to restrict further access and ensure compliance with privacy regulations like GDPR and CCPA. An organization can also do a privacy impact assessment, and regular audits need to be conducted to address any possible privacy issues.
Unknown Risk Factors of the New Technology
AI is a rapidly changing field; unknown risks are associated with it. These AI models are opaque and complex by design, which usually gives way to unpredictable behavior and unwarranted outcomes. For instance, an AI system for predictive maintenance could be devised to foresee equipment failures under normal conditions precisely. Still, it could fail under extreme use cases, where predicting a failure can be very costly.
However, it may make AI inadequate for dealing with brand-new situations, resulting in the wrong decisions. Such unpredictability may be the source of significant operational risks in critical fields, such as health and finance.
Organizations must be judicious in their application of AI and overly emphasize testing and validation. Simulation of various scenarios, even the most improbable ones, can be a solution for exposing the flaws in an AI system. Another way to further mitigate risks regarding the unpredictable behavior of AI is by maintaining a human-in-the-loop approach for AI decisions, wherein human experts review them.
Data Exposure
Data exposure is the real danger associated with AI applications, most of which are machine learning-based. Such systems require significant amounts of data for training and future adaptation. However, mishandling of such data might lead to tremendous security breaches. For instance, using unencrypted data or storing data in unsecured places can expose sensitive information to cyberattacks.
Moreover, AI systems can become potential targets of data breaches. Attackers can utilize AI algorithms’ vulnerabilities to gain unauthorized access to underlying data. For example, input data can be tampered with at either training time or inference time to subvert AI, and this tampering may sometimes reveal sensitive information.
To reduce the risks of data exposure, organizations must put in place robust data security measures. It entails data encryption at rest and in transit by utilizing secure data storage solutions and also, updating security protocols regularly to shield from emerging threats. Strict access controls should also be in place to limit access to sensitive data only to authorized personnel.
Algorithmic Discrimination
Algorithmic bias is one of the leading destructive characteristics found in AI systems. If there is bias in the training data, it can potentially cause bias in the AI model, leading to unfair and discriminatory outcomes. For example, an AI-based model for hiring can be partial to candidates of some demographics in case the training data is just too heavy with successful hiring from that type of demographic. It further leads to the unfolding of already existing biases and bars efforts at promoting diversity and inclusion.
AI bias can also occur and thus entail significant societal consequences. For instance, biased criminal justice algorithms may overtarget some communities, further driving existing inequalities. Likewise, biased healthcare algorithms may result in unequal access to medical treatments between different groups.
Organizations must put fairness and transparency at the core of the development of AI to address algorithmic bias, which calls for working with diversified and representative data sets, regular auditing of bias, and use of fairness metrics as part of AI evaluation. Working with an ethicist and a social scientist can also help identify and mitigate potential biases.
Security Vulnerabilities
AI brings new security challenges to an organization. Adversarial attacks, model inversion attacks, and data poisoning threaten AI systems. Adversarial attacks modify input data to mislead AI models, while a model inversion attack exposes sensitive data by reconstructing it from AI models—data poisoning attacks corrupt AI models by including inappropriate data in training datasets.
These vulnerabilities might even lead to catastrophes, in which data breaches, loss of intellectual property, and compromise in the decision-making process occur. For example, an adversarial attack in the AI system of an autonomous vehicle might trigger wrong decisions in navigation, which in turn might even be harmful to safety.
Organizations can also mitigate security vulnerabilities by implementing a multi-layered security approach. This includes robust security protocol implementation, regular updates of AI models, and security audits to pinpoint and address vulnerabilities. Such techniques include adversarial training, where AI is fundamentally trained both to identify adversarial examples and to consider examples in its prediction.
Unmarked Territory
AI is often deployed in uncharted territory where existing regulations and standards may not provide adequate guidance. This can, in addition, result in legal and ethical challenges. For example, the use of AI in autonomous vehicles will raise questions of liability in the event of road accidents. Correspondingly, AI-based decision-making in health and finance can carry primary ethical considerations.
Furthermore, the more rapidly AI develops, the harder it may be for regulatory bodies to keep up. This may put AI systems in a legal gray area where the hazard of non-compliance rises astronomically, increasing the risk of legal disputes. Take these steps through stakeholder engagement across organizations, regulatory bodies, industry groups, and those interested in deriving and adopting best practices in deploying AI. Participate in the development of industry standards and work collaboratively with policymakers to take part in shaping the current regulatory landscape so AI systems are operating within legal and ethical boundaries.
Compliance Violations
Enterprise organizations need to ensure compliance with regulatory requirements, and AI is bringing new challenges to compliance. For example, AI systems using personal data must comply with data protection regulations such as GDPR and CCPA. Infringement of such rules may result in severe penalties and damage to reputation. Besides this, specific sector regulations may impose additional requirements originating from the nature of the AI systems. For example, there are regulations on algorithmic trading that financial institutions should comply with, and a healthcare provider would want to ensure that an AI-driven diagnostic tool meets medical device regulations. This would mean that organizations put comprehensive governance frameworks around AI to ensure adherence to regulations, which may include regular compliance audits, detailed documentation of AI development and deployment processes, and transparent and explainable AI systems. This ensures that employees are sufficiently trained to meet the requirements set by regulators and a compliance culture that reduces the possibility of failing to comply.
Yet, as much as AI can transform organizations, it is no panacea. The fast adoption of AI without a complete understanding of its risks can bring huge pitfalls. The infiltration of AI into organizations has to be done with a balanced view that sees both its capabilities and vulnerabilities. Addressing privacy concerns, accepting unknown risk factors, preventing data exposure, addressing algorithmic bias, securing against vulnerabilities, navigating unmarked territory, and enabling quantification of compliance are ways in which organizations can harness the potential of AI responsibly and effectively. In essence, this means embedding AI in a way that drives innovation yet keeps the trust and security of stakeholders.